Near-infrared Imaging and Spectroscopy
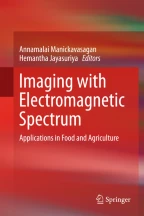
In the electromagnetic spectrum, near infrared (NIR) region covers between 780 nm to 2500 nm. The developments of new NIR techniques like NIR imaging (NIR cameras, NIR hyperspectral imaging systems), Fourier Transform (FT)-NIR spectroscopy, NIR microscopes, and NIR thermal cameras have extended the application of near infrared band dramatically in the last 3 decades, because some of these techniques give spectral as well as spatial data which help to analyze chemical constituents as well as physical and textural parameters of a sample. When an object is illuminated with light, it absorbs, reflects and transmits light at various proportions based on the physical and chemical properties of object. In near infrared imaging systems, this absorbed, transmitted or reflected radiation only at NIR waveband is captured using a NIR detector or sensor. In NIR hyperspectral imaging technique, the object is imaged over a large number of spectral bands and complete reflectance spectrum with spatial (imaging) data are collected. NIR spectroscopy techniques yields only spectral data, and in Fourier transform-near infrared, Fourier transform is applied to convert the raw data into original spectrum. Nowadays, near-infrared imaging and spectroscopy techniques are commercially used for measurement of moisture and other chemical constituents of the cereal grains and oilseeds in grain industry. Meat industry has also started using NIR techniques for non-destructive quality monitoring. The development of multispectral imaging systems based on the indented use, and developments in analysis techniques will help the agricultural and food industry in implementing the NIR imaging and spectroscopy systems for rapid and in-line quality monitoring applications like foreign material detection, discrimination of agricultural and food products based on quality attributes and detection of defects, diseases, and food adulteration.
This is a preview of subscription content, log in via an institution to check access.
Access this chapter
Subscribe and save
Springer+ Basic
€32.70 /Month
- Get 10 units per month
- Download Article/Chapter or eBook
- 1 Unit = 1 Article or 1 Chapter
- Cancel anytime
Buy Now
Price includes VAT (France)
eBook EUR 85.59 Price includes VAT (France)
Softcover Book EUR 105.49 Price includes VAT (France)
Hardcover Book EUR 105.49 Price includes VAT (France)
Tax calculation will be finalised at checkout
Purchases are for personal use only
Similar content being viewed by others
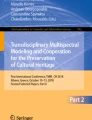
Infrared Hyperspectral Spectroscopic Mapping Imaging from 800 to 5000 nm. A Step Forward in the Field of Infrared “Imaging”
Chapter © 2019
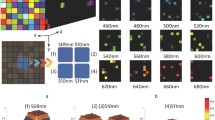
Hyperspectral imaging using the single-pixel Fourier transform technique
Article Open access 24 March 2017
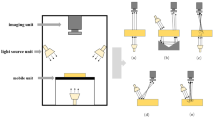
The impact of high-quality data on the assessment results of visible/near-infrared hyperspectral imaging and development direction in the food fields: a review
Article 09 February 2023
References
- Abeni F, Bergoglio G (2001) Characterization of different strains of broiler chicken by carcass measurements, chemical and physical parameters and NIRS on breast muscle. Meat Science 57(2):133–137. doi:http://dx.doi.org/10.1016/S0309-1740(00)00084-X
- Adamopoulos KG, Goula AM, Petropakis HJ (2001) Quality control during processing of feta cheese—NIR application. J Food Compos Anal 14(4):431–440 Google Scholar
- Alomar D, Gallo C, Castañeda M, Fuchslocher R (2003) Chemical and discriminant analysis of bovine meat by near infrared reflectance spectroscopy (NIRS). Meat Sci 63(4):441–450. doi:http://dx.doi.org/10.1016/S0309-1740(02)00101-8
- Anderson S (2007) Determination of fat, moisture, and protein in meat and meat products by using the FOSS FoodScan near-infrared spectrophotometer with FOSS artificial neural network calibration model and associated database: collaborative study. J AOAC Int 90(4):1073–1083 Google Scholar
- Andrés S, Murray I, Navajas EA, Fisher AV, Lambe NR, Bünger L (2007) Prediction of sensory characteristics of lamb meat samples by near infrared reflectance spectroscopy. Meat Sci 76(3):509–516. doi:http://dx.doi.org/10.1016/j.meatsci.2007.01.011
- Ariana D, Lu R (2002) A near-infrared sensing technique for measuring internal quality of apple fruit. Appl Eng Agric 18(5):585–592 Google Scholar
- Ariana DP, Lu R (2008) Quality evaluation of pickling cucumbers using hyperspectral reflectance and transmittance imaging—part II. Performance of a prototype. Sens Instrum Food Qual Saf 2(3):152–160 Google Scholar
- Ariana DP, Lu R, Guyer DE (2006) Near-infrared hyperspectral reflectance imaging for detection of bruises on pickling cucumbers. Comput Electron Agric 53(1):60–70 Google Scholar
- Baer RJ, Frank JF, Loewenstein M, Birth GS (1983) Compositional analysis of whey powders using near infrared diffuse reflectance spectroscopy. J Food Sci 48(3):959–961 Google Scholar
- Baianu I, You T, Costescu D, Lozano P, Prisecaru V, Nelson R (2012) Determination of soybean oil, protein and amino acid residues in soybean seeds by high resolution nuclear magnetic resonance (NMRS) and near Infrared (NIRS). http://dx.doi.org/10.1038/npre.2012.7053.1 Accessed 14 Dec 2012
- Baker JE, Dowell FE, Throne JE (1999) Detection of parasitized rice weevils in wheat kernels with near-infrared spectroscopy. Biol Control 16(1):88–90 Google Scholar
- Barlocco N, Vadell A, Ballesteros F, Galietta G, Cozzolino D (2006) Predicting intramuscular fat, moisture and Warner-Bratzler shear force in pork muscle using near infrared reflectance spectroscopy. Anim Sci 82(1):111–116 Google Scholar
- Ben-Gera I, Norris KH (1968a) Determination of moisture content in soybeans by direct spectrophotometry. Isr J Agric Res 18(3):125–132 Google Scholar
- Ben-Gera I, Norris KH (1968b) Direct spectrophotometric determination of fat and moisture in meat products. J Food Sci 33(1):64–67. doi:10.1111/j.1365-2621.1968.tb00885.xGoogle Scholar
- Ben-Gera I, Norris KH (1968c) Influence of fat concentration on the absorption spectrum of milk in the near-infrared region. Isr J Agric Res 18(3):117–124 Google Scholar
- Berardo N, Brenna O, Amato A, Valoti P, Pisacane V, Motto M (2004) Carotenoids concentration among maize genotypes measured by near infrared reflectance spectroscopy (NIRS). Innovative Food Sci Emerg Technol 5(3):393–398 Google Scholar
- Bertrand D, Robert P, Loisel W (1985) Identification of some wheat varieties by near infrared reflectance spectroscopy. J Sci Food Agric 36(11):1120–1124 Google Scholar
- Berzaghi P, Dalle Zotte A, Jansson LM, Andrighetto I (2005) Near-infrared reflectance spectroscopy as a method to predict chemical composition of breast meat and discriminate between different n-3 feeding sources. Poult Sci 84(1):128–136 Google Scholar
- Brøndum J, Munck L, Henckel P, Karlsson A, Tornberg E, Engelsen SB (2000) Prediction of water-holding capacity and composition of porcine meat by comparative spectroscopy. Meat Sci 55(2):177–185. doi:http://dx.doi.org/10.1016/S0309-1740(99)00141-2
- Byrne CE, Downey G, Troy DJ, Buckley DJ (1998) Non-destructive prediction of selected quality attributes of beef by near-infrared reflectance spectroscopy between 750 and 1098 nm. Meat Sci 49(4):399–409. doi:http://dx.doi.org/10.1016/S0309-1740(98)00005-9
- Call J, Lodder RA (2002) Application of a liquid crystal tunable filter to near-infrared spectral searches. Proc SETICon 02:18–22 Google Scholar
- Cattaneo TMP, Giardina C, Sinelli N, Riva M, Giangiacomo R (2005) Application of FT-NIR and FT-IR spectroscopy to study the shelf-life of Crescenza cheese. Int Dairy J 15(6):693–700 Google Scholar
- Chan DE, Walker PN, Mills EW (2002) Prediction of pork quality characteristics using visible and near-infrared spectroscopy. Trans ASAE 45(5):1519–1527 Google Scholar
- Chau A, Whitworth M, Leadley C, Millar S (2009) Innovative sensors to rapidly and non-destructively determine fish freshness. Seafish Industrial Authority Google Scholar
- Cheng X, Chen YR, Tao Y, Wang CY, Kim MS, Lefcourt AM (2004) A novel integrated PCA and FLD method on hyperspectral image feature extraction for cucumber chilling damage inspection. Trans ASAE 47(4):1313–1320 Google Scholar
- Choudhary R, Mahesh S, Paliwal J, Jayas DS (2009) Identification of wheat classes using wavelet features from near infrared hyperspectral images of bulk samples. Biosyst Eng 102(2):115–127 Google Scholar
- Cocchi L, Vescovi L, Petrini LE, Petrini O (2006) Heavy metals in edible mushrooms in Italy. Food Chem 98(2):277–284 Google Scholar
- Cogdill RP, Hurburgh CR, Rippke GR (2004) Single-kernel maize analysis by near-infrared hyperspectral imaging. Trans ASAE 47(1):311–320 Google Scholar
- Contal L, Leon V, Downey G (2002) Detection and quantification of apple adulteration in strawberry and raspberry purées using visible and near infrared spectroscopy. J Near Infrared Spectrosc 10(4):289–300 Google Scholar
- Cozzolino D, Barlocco N, Vadell A, Ballesteros F, Gallieta G (2003) The use of visible and near-infrared reflectance spectroscopy to predict colour on both intact and homogenised pork muscle. LWT—Food Science and Technology 36(2):195–202. doi:http://dx.doi.org/10.1016/S0023-6438(02)00199-8
- Cozzolino D, Murray I (2002) Effect of sample presentation and animal muscle species on the analysis of meat by near infrared reflectance spectroscopy. J Near Infrared Spectrosc 10(1):37–44 Google Scholar
- Cozzolino D, Murray I, Scaife J, Paterson R (2000) Study of dissected lamb muscles by visible and near infrared reflectance spectroscopy for composition assessment. Anim Sci 70(3):417–423 Google Scholar
- De Marchi M, Berzaghi P, Boukha A, Mirisola M, Gallo L (2010) Use of near infrared spectroscopy for assessment of beef quality traits. Ital J Anim Sci 6(1):421–423 Google Scholar
- Delwiche SR (1995) Single wheat kernel analysis by near-infrared transmittance: protein content. Cereal Chem 72(1):11–16 Google Scholar
- Delwiche SR (1998) Protein content of single kernels of wheat by near-infrared reflectance spectroscopy. J Cereal Sci 27(3):241–254 Google Scholar
- Delwiche SR (2003) Classification of scab-and other mold-damaged wheat kernels by near-infrared reflectance spectroscopy. Trans ASAE 46(3):731–738 Google Scholar
- Delwiche SR, Chen Y-R, Hruschka WR (1995) Differentiation of hard red wheat by near-infrared analysis of bulk samples. Cereal Chem 72(3):243–247 Google Scholar
- Delwiche SR, Graybosch RA (2002) Identification of waxy wheat by near-infrared reflectance spectroscopy. J Cereal Sci 35(1):29–38 Google Scholar
- Delwiche SR, Massie DR (1996) Classification of wheat by visible and near-infrared reflectance from single kernels. Cereal Chem 73(3):399–405 Google Scholar
- Delwiche SR, Norris KH (1993) Classification of hard red wheat by near-infrared diffuse reflectance spectroscopy. Cereal Chem 70(1):29 Google Scholar
- Ding H, Xu RJ, Chan DKO (1999) Identification of broiler chicken meat using a visible/near-infrared spectroscopic technique. J Sci Food Agric 79(11):1382–1388 Google Scholar
- Ding HB, Xu RJ (1999) Differentiation of beef and kangaroo meat by visible/near-infrared reflectance spectroscopy. J Food Sci 64(5):814–817. doi:10.1111/j.1365-2621.1999.tb15918.xGoogle Scholar
- Ding HB, Xu RJ (2000) Near-infrared spectroscopic technique for detection of beef hamburger Adulteration. J Agric Food Chem 48(6):2193–2198. doi:10.1021/jf9907182Google Scholar
- Dowell FE (1997) Effect of NaOH on visible wavelength spectra of single wheat kernels and color classification efficiency. Cereal Chem 74(5):617–620 Google Scholar
- Dowell FE (2000) Differentiating vitreous and nonvitreous durum wheat kernels by using near-infrared spectroscopy. Cereal Chem 77(2):155–158 MathSciNetGoogle Scholar
- Dowell FE, Pearson TC, Maghirang EB, Xie F, Wicklow DT (2002) Reflectance and transmittance spectroscopy applied to detecting fumonisin in single corn kernels infected with Fusarium verticillioides. Cereal Chem 79(2):222–226 Google Scholar
- Dowell FE, Throne JE, Baker JE (1998) Automated nondestructive detection of internal insect infestation of wheat kernels by using near-infrared reflectance spectroscopy. J Econ Entomol 91(4):899–904 Google Scholar
- Downey G (1986) Development, evaluation and collaborative testing of calibrations for the prediction of protein and moisture in ground barley by near infra-red reflectance. Ir J Food Sci Technol 10:119–126 Google Scholar
- Downey G, Robert P, Bertrand D, Kelly PM (1990) Classification of commercial skim milk powders according to heat treatment using factorial discriminant analysis of near-infrared reflectance spectra. Appl Spectrosc 44(1):150–155 Google Scholar
- Ellis JW, Bath J (1938) Modifications in the near infra-red absorption spectra of protein and of light and heavy water molecules when water is bound to gelatin. J Chem Phys 6(11):723–729 Google Scholar
- ElMasry G, Barbin DF, Sun DW, Allen P (2012) Meat quality evaluation by hyperspectral imaging technique: an overview. Crit Rev Food Sci Nutr 52(8):689–711. doi:10.1080/10408398.2010.507908Google Scholar
- ElMasry G, Wang N, ElSayed A, Ngadi M (2007) Hyperspectral imaging for nondestructive determination of some quality attributes for strawberry. J Food Eng 81(1):98–107 Google Scholar
- ElMasry G, Wold JP (2008) High-speed assessment of fat and water content distribution in fish fillets using online imaging spectroscopy. J Agric Food Chem 56(17):7672–7677 Google Scholar
- Evans D, Scotter C, Day L, Hall M (1993) Determination of the authenticity of orange juice by discriminant analysis of near infrared spectra. J Near Infrared Spectrosc 1:33–44 Google Scholar
- Frank JF, Birth GS (1982) Application of near infrared reflectance spectroscopy to cheese analysis. J Dairy Sci 65(7):1110–1116 Google Scholar
- Frankhuizen R (1992) NIR analysis of dairy products. Pract Spectrosc Ser 13:609 Google Scholar
- Gaitán-Jurado AJ, Ortiz-Somovilla V, España-España F, Pérez-Aparicio J, De Pedro-Sanz EJ (2008) Quantitative analysis of pork dry-cured sausages to quality control by NIR spectroscopy. Meat Sci 78(4):391–399 Google Scholar
- Gangidi RR, Proctor A, Pohlman FW, Meullenet J-F (2005) Rapid determination of spinal cord content in ground beef by near-infrared spectroscopy. J Food Sci 70(6):c397–c400. doi:10.1111/j.1365-2621.2005.tb11436.xGoogle Scholar
- Garcia-Rey RM, Garcia-Olmo J, De Pedro E, Quiles-Zafra R, de Castro Luque MD (2005) Prediction of texture and colour of dry-cured ham by visible and near infrared spectroscopy using a fiber optic probe. Meat Sci 70(2):357–363 Google Scholar
- Gat N (2000) Imaging spectroscopy using tunable filters: a review. In: AeroSense 2000. International society for optics and photonics, pp 50–64 Google Scholar
- Giangiacomo R, Braga F, Galliena C (1991) Use of near-infrared spectroscopy to detect whey powder mixed with milk powder. In: Murray I, Cowe IA (eds) Making light work: advances in near-infrared spectroscopy. VCH, Weinheim, pp 399–407 Google Scholar
- Gorretta N, Roger JM, Aubert M, Bellon-Maurel V, Campan F, Roumet P (2006) Determining vitreousness of durum wheat kernels using near infrared hyperspectral imaging. J Near Infrared Spectrosc 14(4):231–239 Google Scholar
- Goulden JDS (1957) 676. Diffuse reflexion spectra of dairy products in the near infra-red region. J Dairy Res 24(02):242–251. doi:10.1017/S0022029900008785Google Scholar
- Gowen AA, O’Donnell CP, Cullen PJ, Downey G, Frias JM (2007) Hyperspectral imaging—an emerging process analytical tool for food quality and safety control. Trends Food Sci Technol 18(12):590–598 Google Scholar
- Gowen AA, Taghizadeh M, O’Donnell CP (2009) Identification of mushrooms subjected to freeze damage using hyperspectral imaging. J Food Eng 93(1):7–12 Google Scholar
- Grau R, Sánchez AJ, Girón J, Iborra E, Fuentes A, Barat JM (2011) Nondestructive assessment of freshness in packaged sliced chicken breasts using SW-NIR spectroscopy. Food Res Int 44(1):331–337 Google Scholar
- Gributs CEW, Burns DH (2006) Parsimonious calibration models for near-infrared spectroscopy using wavelets and scaling functions. Chemometr Intell Lab Syst 83(1):44–53 Google Scholar
- Guy RCE, Osborne BG, Robert P (1996) The application of near infrared reflectance spectroscopy to measure the degree of processing in extrusion cooking processes. J Food Eng 27(3):241–258 Google Scholar
- Hareland GA (1994) Evaluation of flour particle size distribution by laser diffraction, sieve analysis and near-infrared reflectance spectroscopy. J Cereal Sci 20(2):183–190 Google Scholar
- Hart JR, Norris KH, Golumbic C (1962) Determination of the moisture content of seeds by near-infrared spectrophotometry of their methanol extracts. Cereal Chem 39(2):94–99 Google Scholar
- Headwall (2012) Spectral imaging capabilities of hyperspec™ imaging technology in pharmaceutical operations. http://www.headwallphotonics.com/downloads/hw_hyperspectral-in-pharma.pdf. Accessed 12 Dec 2012
- Hildrum KI, Nilsen BN, Westad F, Wahlgren NM (2004) In-line analysis of ground beef using a diode array near infrared instrument on a conveyor belt. J Near Infrared Spectrosc 12:367–376 Google Scholar
- Hindle PH (2008) Historical development. In: Burns DA, Ciurczak EW (eds) Handbook of near-infrared analysis, vol 35. CRC, Boca Raton, pp 3–6 Google Scholar
- Hoving-Bolink AH, Vedder HW, Merks JWM, de Klein WJH, Reimert HGM, Frankhuizen R, van den Broek WHAM, Lambooij eE (2005) Perspective of NIRS measurements early post mortem for prediction of pork quality. Meat Sci 69(3):417–423. doi:http://dx.doi.org/10.1016/j.meatsci.2004.08.012
- Hruschka WR (1987) Data analysis: wavelength selection methods. In: Williams P, Norris KH (eds) Near-infrared technology in the agricultural and food industries, vol 2., AACCSt. Paul, Minnesota, pp 39–58 Google Scholar
- Jayas DS, Singh CB, Paliwal J (2010) Classification of wheat kernels using near-infrared reflectance hyperspectral imaging. In: Sun D-W (ed) Hyperspectral imaging for food quality analysis and control, 1st edn. Academic Press, London, pp 449–470 Google Scholar
- Jeyamkondan S, Kranzler GA, Morgan BJ, Rust S (2003) Predicting beef tenderness using near-infrared spectroscopy. Proc SPIE 2003:356–365 Google Scholar
- Kamishikiryo-Yamashita H, Oritani Y, Takamura H, Matoba T (1994) Protein content in milk by near-infrared spectroscopy. J Food Sci 59(2):313–315 Google Scholar
- Kamruzzaman M, ElMasry G, Sun D-W, Allen P (2011) Application of NIR hyperspectral imaging for discrimination of lamb muscles. J Food Eng 104(3):332–340. doi:http://dx.doi.org/10.1016/j.jfoodeng.2010.12.024
- Kamruzzaman M, Sun D-W, ElMasry G, Allen P (2012) Fast detection and visualization of minced lamb meat adulteration using NIR hyperspectral imaging and multivariate image analysis. Talanta 103:130–136 Google Scholar
- Kaye W (1954) Near-infrared spectroscopy: I. Spectral identification and analytical applications. Spectrochim Acta 6(4):257–287. doi:http://dx.doi.org/10.1016/0371-1951(54)80011-7
- Kim MS, Chen YR, Mehl PM (2001) Hyperspectral reflectance and fluorescence imaging system for food quality and safety. Trans ASAE 44(3):721–729 Google Scholar
- Kim MS, Lefcourt AM, Chao K, Chen YR, Kim I, Chan DE (2002) Multispectral detection of fecal contamination on apples based on hyperspectral imagery: part I. Application of visible and near-infrared reflectance imaging. Trans ASAE 45(6):2027–2038 Google Scholar
- Lawrence KC, Windham WR, Park B, Smith DP, Poole GH (2003) Comparison between visible/NIR spectroscopy and hyperspectral imaging for detecting surface contaminants on poultry carcasses. In: Conference on monitoring food safety, Agriculture, and Plant Health. Providence, Rhode Islands, pp 35–42 Google Scholar
- Lee K-J, Kang S, Kim MS, Noh SH (2005). Hyperspectral imaging for detecting defect on apples. In: 2005 ASAE annual international meeting, Tampa, Florida, Paper no 053075, 17–20 July 2005 Google Scholar
- Lefcout AM, Kim MS, Chen Y-R, Kang S (2006) Systematic approach for using hyperspectral imaging data to develop multispectral imagining systems: detection of feces on apples. Comput Electron Agric 54(1):22–35 Google Scholar
- León L, Kelly JD, Downey G (2005) Detection of apple juice adulteration using near-infrared transflectance spectroscopy. Appl Spectrosc 59(5):593–599 Google Scholar
- Liu Y, Chen Y-R, Kim MS, Chan DE, Lefcourt AM (2007) Development of simple algorithms for the detection of fecal contaminants on apples from visible/near infrared hyperspectral reflectance imaging. J Food Eng 81(2):412–418 Google Scholar
- Liu Y, Lyon BG, Windham WR, Lyon CE, Savage EM (2004) Prediction of physical, color, and sensory characteristics of broiler breasts by visible/near infrared reflectance spectroscopy. Poult Sci 83(8):1467–1474 Google Scholar
- Lu R (2003) Detection of bruises on apples using near-infrared hyperspectral imaging. Trans ASAE 46(2):523–530 Google Scholar
- Lu R, Peng Y (2006) Hyperspectral scattering for assessing peach fruit firmness. Biosyst Eng 93(2):161–171 MathSciNetGoogle Scholar
- Maghirang EB, Dowell FE (2003) Hardness measurement of bulk wheat by single-kernel visible and near-infrared reflectance spectroscopy. Cereal Chem 80(3):316–322 Google Scholar
- Maghirang EB, Dowell FE, Baker JE, Throne JE (2003) Automated detection of single wheat kernels containing live or dead insects using near-infrared reflectance spectroscopy. Trans ASAE 46(4):1277–1284 Google Scholar
- Mahesh S, Jayas DS, Paliwal J, White NDG (2011) Identification of wheat classes at different moisture levels using near-infrared hyperspectral images of bulk samples. Sens Instrum Food Qual Saf 5(1):1–9 Google Scholar
- Mahesh S, Manickavasagan A, Jayas DS, Paliwal J, White NDG (2008) Feasibility of near-infrared hyperspectral imaging to differentiate Canadian wheat classes. Biosyst Eng 101(1):50–57 Google Scholar
- Manickavasagan A, Ganeshmoorthy K (2013) Total soluble solid (TSS) measurement in dates at tamr stage using NIR reflectance imaging. In: CSBE/SCGAB 2013 annual conference, Sakatoon, SK, Canada. Paper No. CSBE13-003, 7–10 July 2013 Google Scholar
- Manley M, Downey G, Baeten V (2008) Spectroscopic technique: near-infrared (NIR) spectroscopy. In: Sun DW (ed) Modern Techniques for Food Authentication, 1st edn. Academic Press, New York, pp 65–115 Google Scholar
- Maraboli A, Cattaneo TMP, Giangiacomo R (2002) Detection of vegetable proteins from soy, pea and wheat isolates in milk powder by near infrared spectroscopy. J Near Infrared Spectrosc 10(1):63–70 Google Scholar
- McCarthy WJ, Kemeny GJ (2008) Fourier transform spectrophotometers in the near-infrared. Pract Spectrosc Ser 35:79 Google Scholar
- McClure WF (2003) 204 years of near infrared technology: 1800–2003. J Near Infrared Spectrosc 11(6):487–518 Google Scholar
- McDevitt RM, Gavin AJ, Andrés S, Murray I (2005) The ability of visible and near infrared reflectance spectroscopy to predict the chemical composition of ground chicken carcasses and to discriminatebetween carcasses from different genotypes. J Near Infrared Spectrosc 13(3):109–117 Google Scholar
- McElhinney J, Downey G, O’Donnell C (1999) Quantitation of lamb content in mixtures with raw minced beef using visible, near and mid-infrared spectroscopy. J Food Sci 64(4):587–591 Google Scholar
- Mehl PM, Chen Y-R, Kim MS, Chan DE (2004) Development of hyperspectral imaging technique for the detection of apple surface defects and contaminations. J Food Eng 61(1):67–81 Google Scholar
- Meulemans A, Dotreppe O, Leroy B, Istasse L, Clinquart A (2002) Prediction of organoleptic and technological characteristics of pork meat by near infrared spectroscopy. Sci des Aliments 23:159–162 Google Scholar
- Meullenet J-F, Jonville E, Grezes D, Owens CM (2004) Prediction of the texture of cooked poultry pectoralis major muscles by near-infrared reflectance analysis of raw meat. J Texture Stud 35(6):573–585. doi:10.1111/j.1538-7836.2004.01165.x-i1Google Scholar
- Miller CE (2001) Chemical principles of near infrared technology. In: Williams P, Norris K (eds) Near infrared technology in the agricultural and food industries. American Association of Cereal Chemists Inc, St. Paul, Minnesota, pp 19–37 Google Scholar
- Miralbés C (2004) Quality control in the milling industry using near infrared transmittance spectroscopy. Food Chem 88(4):621–628 Google Scholar
- Mitsumoto M, Maeda S, Mitsuhashi T, Ozawa S (1991) Near-Infrared spectroscopy determination of physical and chemical characteristics in beef cuts. J Food Sci 56(6):1493–1496 Google Scholar
- Mohan LA, Karunakaran C, Jayas DS, White NDG (2005) Classification of bulk cereals using visible and NIR reflectance characteristics. Can Biosyst Eng 47(7):7–14 Google Scholar
- Murray I, Williams PC (1987) Chemical principles of near-infrared technology. In: Williams P, Norris K (eds) Near infrared technology in the agricultural and food industries, 1st edn. American Association of Cereal Chemists Inc, St. Paul, Minnesota Google Scholar
- Naganathan GK, Grimes LM, Subbiah J, Calkins CR, Samal A, Meyer GE (2008a) Partial least squares analysis of near-infrared hyperspectral images for beef tenderness prediction. Sens Instrum Food Qual Saf 2(3):178–188 Google Scholar
- Naganathan GK, Grimes LM, Subbiah J, Calkins CR, Samal A, Meyer GE (2008b) Visible/near-infrared hyperspectral imaging for beef tenderness prediction. Comput Electron Agric 64(2):225–233 Google Scholar
- Nagata M, Tallada JG, Kobayashi T, Cui Y, Gejima Y (2004) Predicting maturity quality parameters of strawberries using hyperspectral imaging. In: Paper presented at the 2004 ASAE/CSAE annual international meeting, Ottowa, Ontario, 1–4 Aug 2004 Google Scholar
- Nagata M, Tallada JG, Kobayashi T, Toyoda H (2005) NIR hyperspectral imaging for measurement of internal quality in strawberries. In: Paper presented at the 2005 ASAE annual international meeting, Tampa, Florida, 17–20 July 2005 Google Scholar
- Nakariyakul S, Casasent DP (2008) Hyperspectral waveband selection for contaminant detection on poultry carcasses. Opt Eng 47(8):087202–087209 Google Scholar
- Nicolaï BM, Lötze E, Peirs A, Scheerlinck N, Theron KI (2006) Non-destructive measurement of bitter pit in apple fruit using NIR hyperspectral imaging. Postharvest Biol Technol 40(1):1–6 Google Scholar
- Noh HK, Lu R (2007) Hyperspectral laser-induced fluorescence imaging for assessing apple fruit quality. Postharvest Biol Technol 43(2):193–201 Google Scholar
- Norris KH, Barnes RF, Moore JE, Shenk JS (1976) Predicting forage quality by infrared reflectance spectroscopy. J Anim Sci 43(4):889–897 Google Scholar
- Norris KH, Hart JR (1965) Direct spectroscopic determination of moisture content of grain and seeds. In: International symposium on humidity and moisture, Washington, 1963. Reinhold New York Google Scholar
- Ortiz-Somovilla V, España-España F, Gaitán-Jurado AJ, Pérez-Aparicio J, De Pedro-Sanz EJ (2007) Proximate analysis of homogenized and minced mass of pork sausages by NIRS. Food Chem 101(3):1031–1040. doi:http://dx.doi.org/10.1016/j.foodchem.2006.02.058
- Osborne BG, Fearn T, Hindle PH (1993) Theory of near-infrared spectrometry. In: Osborne B, Fearn T, Hindle P (eds) Near infrared spectroscopy in food analysis. Longman Singapore Publishers, Singapore Google Scholar
- Paliwal J, Wang W, Symons SJ, Karunakaran C (2004) Insect species and infestation level determination in stored wheat using near-infrared spectroscopy. Can Biosyst Eng 46(7):17–24 Google Scholar
- Panford JA, Deman JM (1990) Determination of oil content of seeds by NIR: influence of fatty acid composition on wavelength selection. J Am Oil Chem Soc 67(8):473–482 Google Scholar
- Park B, Chen YR, Hruschka WR, Shackelford SD, Koohmaraie M (1998) Near-infrared reflectance analysis for predicting beef longissimus tenderness. J Anim Sci 76(8):2115–2120 Google Scholar
- Pearson TC, Wicklow DT, Maghirang EB, Xie F, Dowell FE (2001) Detecting aflatoxin in single corn kernels by transmittance and reflectance spectroscopy. Trans ASAE 44(5):1247–1254 Google Scholar
- Pedretti N, Bertrand D, Semenou M, Robert P, Giangiacomo R (1993) Application of an experimental design to the detection of foreign substances in milk. J Near Infrared Spectrosc 1:174–184 Google Scholar
- Peirs A, Scheerlinck N, De Baerdemaeker J, Nicolai BM (2003) Starch index determination of apple fruit by means of a hyperspectral near infrared reflectance imaging system. J Near Infrared Spectrosc 11(5):379–390 Google Scholar
- Peiris KHS, Pumphery MO, Dowell FE (2009) NIR absorbance characteristics of deoxynivalenol and of sound and fusarium—damaged wheat kernels. J Near Infrared Spectrosc 17(4):213–221 Google Scholar
- Peng Y, Wu J (2008) Hyperspectral scattering profiles for prediction of beef tenderness. In: Paper presented at the 2008 ASABE annual international meeting, Providence, Rhode Island Google Scholar
- Peng Y, Zhang J, Wu J, Hang H, Kim M, Tu S, Chao K (2009) Hyperspectral scattering profiles for prediction of the microbial spoilage of beef. In: Sensing for agriculture and food quality and safety, Orlando, Florida 2009. SPIE, Bellingham, Washington pp Q73150–Q73112 Google Scholar
- Perez-Mendoza J, Throne JE, Dowell FE, Baker JE (2003) Detection of insect fragments in wheat flour by near-infrared spectroscopy. J Stored Prod Res 39(3):305–312 Google Scholar
- Pettersson H, Åberg L (2003) Near infrared spectroscopy for determination of mycotoxins in cereals. Food Control 14(4):229–232 Google Scholar
- Pierce MM, Wehling RL (1994) Comparison of sample handling and data treatment methods for determining moisture and fat in Cheddar cheese by near-infrared spectroscopy. J Agric Food Chem 42(12):2830–2835 Google Scholar
- Pillonel L, Luginbühl W, Picque D, Schaller E, Tabacchi R, Bosset J (2003) Analytical methods for the determination of the geographic origin of Emmental cheese: mid-and near-infrared spectroscopy. Eur Food Res Technol 216(2):174–178 Google Scholar
- Polder G, Van Der Heijden GWA, Waalwijk C, Young IT (2005) Detection of Fusarium in single wheat kernels using spectral imaging. Sci Technol 33(3):655–668 Google Scholar
- Prieto N, Andrés S, Giráldez FJ, Mantecón AR, Lavín P (2006) Potential use of near infrared reflectance spectroscopy (NIRS) for the estimation of chemical composition of oxen meat samples. Meat Sci 74(3):487–496. doi:http://dx.doi.org/10.1016/j.meatsci.2006.04.030
- Prieto N, Andrés S, Giráldez FJ, Mantecón AR, Lavín P (2008) Ability of near infrared reflectance spectroscopy (NIRS) to estimate physical parameters of adult steers (oxen) and young cattle meat samples. Meat Sci 79(4):692–699. doi:http://dx.doi.org/10.1016/j.meatsci.2007.10.035
- Prieto N, Roehe R, Lavín P, Batten G, Andrés S (2009) Application of near infrared reflectance spectroscopy to predict meat and meat products quality: a review. Meat Sci 83(2):175–186 Google Scholar
- Qiao J, Ngadi MO, Wang N, Gariépy C, Prasher SO (2007) Pork quality and marbling level assessment using a hyperspectral imaging system. J Food Eng 83(1):10–16 Google Scholar
- Rahim HA, Ghazali R (2012) The application of near-infrared spectroscopy for poultry meat grading. In: 2012 IEEE 8th international colloquium on signal processing and its applications (CSPA), Melaka, Malaysia, pp 58–62 Google Scholar
- Rannou H, Downey G (1997) Discrimination of raw pork, chicken and turkey meat by spectroscopy in the visible, near-and mid-infrared ranges. Anal Commun 34(12):401–404 Google Scholar
- Reid LM, Woodcock T, O’Donnell CP, Kelly JD, Downey G (2005) Differentiation of apple juice samples on the basis of heat treatment and variety using chemometric analysis of MIR and NIR data. Food Res Int 38(10):1109–1115 Google Scholar
- Ridgway C, Chambers J (1998) Detection of insects inside wheat kernels by NIR imaging. J Near Infrared Spectrosc 6(1):115–120 Google Scholar
- Ridgway C, Chambers J, Cowe IA (1999) Detection of grain weevils inside single wheat kernels by a very near infrared two-wavelength model. J Near Infrared Spectrosc 7(4):213–222 Google Scholar
- Riovanto R, De Marchi M, Cassandro M, Penasa M (2012) Use of near infrared transmittance spectroscopy to predict fatty acid composition of chicken meat. Food Chem 134(4):2459–2464. doi:http://dx.doi.org/10.1016/j.foodchem.2012.04.038
- Ripoll G, Albertí P, Panea B, Olleta JL, Sañudo C (2008) Near-infrared reflectance spectroscopy for predicting chemical, instrumental and sensory quality of beef. Meat Sci 80(3):697–702. doi:http://dx.doi.org/10.1016/j.meatsci.2008.03.009
- Robert P, Bertrand D, Devaux MF, Grappin R (1987) Multivariate analysis applied to near-infrared spectra of milk. Anal Chem 59(17):2187–2191 Google Scholar
- Rødbotten R, Mevik B-H, Hildrum KI (2001) Prediction and classification of tenderness in beef from non-invasive diode array detected NIR spectra. J Near Infrared Spectrosc 9(3):199–210 Google Scholar
- Rødbotten R, Nilsen BN, Hildrum KI (2000) Prediction of beef quality attributes from early post mortem near infrared reflectance spectra. Food Chem 69(4):427–436 doi:http://dx.doi.org/10.1016/S0308-8146(00)00059-5
- Rodriguez-Otero JL, Hermida M, Centeno J (1997) Analysis of dairy products by near-infrared spectroscopy: a review. J Agric Food Chem 45(8):2815–2819 Google Scholar
- Ruan R (2002) Non-destructive determination of deoxynivalenol levels in barley using near-infrared spectroscopy. Appl Eng Agric 18(5):549–554 Google Scholar
- Ruan R, Li Y, Lin X, Chen P (2002) Non-destructive determination of deoxynivalenol levels in barley using near-infrared spectroscopy. Appl Eng Agric 18(5):549–553 Google Scholar
- Sanderson R, Lister SJ, Dhanoa MS, Barnes RJ, Thomas C (1997) Use of near infrared reflectance spectroscopy to predict and compare the composition of carcass samples from young steers. Anim Sci 65(01):45–54. doi:10.1017/S1357729800016283Google Scholar
- Sato T, Kawano S, Iwamoto M (1990) Detection of foreign fat adulteration of milk fat by near infrared spectroscopic method. J Dairy Sci 73(12):3408–3413 Google Scholar
- Scotter CNG, Legrand A (1995) Near-infrared (NIR) spectroscopy as a screening technique for fruit juice verification. Fruit Process 5:255–260 Google Scholar
- Segtnan VH, Høy M, Sørheim O, Kohler A, Lundby F, Wold JP, Ofstad R (2009) Noncontact salt and fat distributional analysis in salted and smoked salmon fillets using X-ray computed tomography and NIR interactance imaging. J Agric Food Chem 57(5):1705–1710 Google Scholar
- Senthilkumar T, Singh CB, Jayas DS, White NDG (2012) Detection of fungal infection in canola using near-infrared hyperspectral imaging. J Agric Eng 49(1):21–27 Google Scholar
- Shahin M, Symons S (2008) Detection of hard vitreous and starchy kernels in amber durum wheat samples using hyperspectral imaging. NIR News 19(5):16–18 Google Scholar
- Shilton N, Downey G, McNulty P (1998) Detection of orange juice adulteration by near-infrared spectroscopy. Seminars in food analysis, 1998. Chapman & Hall, London, pp 155–162 Google Scholar
- Singh C, Jayas DS, Paliwal J, White N (2009a) Detection of insect-damaged wheat kernels using near-infrared hyperspectral imaging. J Stored Prod Res 45(3):151–158 Google Scholar
- Singh CB, Jayas DS, Paliwal J, White NDG (2009b) Detection of sprouted and midge-damaged wheat kernels using near-infrared hyperspectral imaging. Cereal Chem 86(3):256–260 Google Scholar
- Slaughter DC, Norris KH, Hruschka WR (1992) Quality and classification of hard red wheat. Cereal Chem 69(4):7423–7432 Google Scholar
- Thyholt K, Isaksson T (1997) Differentiation of frozen and unfrozen beef using near-infrared spectroscopy. J Sci Food Agric 73(4):525–532. doi:10.1002/(sici)1097-0010(199704)73:43.0.co;2-cGoogle Scholar
- Tøgersen G, Isaksson T, Nilsen BN, Bakker EA, Hildrum KI (1999) On-line NIR analysis of fat, water and protein in industrial scale ground meat batches. Meat Sci 51(1):97–102. doi:http://dx.doi.org/10.1016/S0309-1740(98)00106-5
- Tran CD (2003) Infrared multispectral imaging: principles and instrumentation. Appl Spectrosc Rev 38(2):133–153 Google Scholar
- Twomey M, Downey G, McNulty PB (2006) The potential of NIR spectroscopy for the detection of the adulteration of orange juice. J Sci Food Agric 67(1):77–84 Google Scholar
- Venel C, Mullen AM, Downey G, Troy D (2001) Prediction of tenderness and other quality attributes of beef by near infrared reflectance spectroscopy between 750 and 1100 nm; further studies. J Near Infrared Spectrosc 9(3):185–198 Google Scholar
- Viljoen M, Hoffman L, Brand T (2005) Prediction of the chemical composition of freeze dried ostrich meat with near infrared reflectance spectroscopy. Meat Sci 69(2):255–261. doi:http://dx.doi.org/10.1016/j.meatsci.2004.07.008
- Viljoen M, Hoffman L, Brand T (2007) Prediction of the chemical composition of mutton with near infrared reflectance spectroscopy. Small Ruminant Res 69(1):88–94 Google Scholar
- Wang D, Dowell FE, Chung DS (2001) Assessment of heat-damaged wheat kernels using near-infrared spectroscopy. Cereal Chem 78(5):625–628 Google Scholar
- Wang D, Dowell FE, Dempster R (2002) Determining vitreous subclasses of hard red spring wheat using visible/near-infrared spectroscopy. Cereal Chem 79(3):418–422 Google Scholar
- Wang D, Dowell FE, Ram MS, Schapaugh WT (2004a) Classification of fungal-damaged soybean seeds using near-infrared spectroscopy. Int J Food Prop 7(1):75–82 Google Scholar
- Wang W, Pailwal J, Jayas DS (2004) Determination of moisture content of ground wheat using near-infrared spectroscopy. In: Paper presented at the 2004 ASAE/CSAE annual international conference, Ottowa, Ontario, 1–4 Aug 2004 Google Scholar
- Wang W, Paliwal J (2007) Near-infrared spectroscopy and imaging in food quality and safety. Sens Instrum Food Qual Saf 1(4):193–207 MATHGoogle Scholar
- Wesley IJ, Larroque O, Osborne BG, Azudin N, Allen H, Skerritt JH (2001) Measurement of gliadin and glutenin content of flour by NIR spectroscopy. J Cereal Sci 34(2):125–133 Google Scholar
- Williams P, Geladi P, Fox G, Manley M (2009) Maize kernel hardness classification by near infrared (NIR) hyperspectral imaging and multivariate data analysis. Anal Chim Acta 653(2):121–130 Google Scholar
- Workman JJ, Burns DA (2001) Commercial NIR instrumentation. Pract Spectrosc Ser 27:53–70 Google Scholar
- Xing J, Bravo C, Jancsók PT, Ramon H, De Baerdemaeker J (2005) Detecting bruises on ‘Golden Delicious’ apples using hyperspectral imaging with multiple wavebands. Biosyst Eng 90(1):27–36 Google Scholar
- Xing J, Van Hung P, Symons S, Shahin M, Hatcher D (2009) Using a short wavelength infrared (SWIR) hyperspectral imaging system to predict alpha amylase activity in individual Canadian western wheat kernels. Sens Instrum Food Qual Saf 3(4):211–218 Google Scholar
- Yang C-C, Chao K, Kim MS (2009) Machine vision system for online inspection of freshly slaughtered chickens. Sens Instrum Food Qual Saf 3(1):70–80 Google Scholar
- Yoon SC, Lawrence KC, Smith DP, Park B, Windham WR (2006) Bone fragment detection in chicken breast fillets using diffuse scattering patterns of back-illuminated structured light. In: Optics East 2006. International society for optics and photonics, pp 63810G–63810G Google Scholar
- Yoon SC, Lawrence KC, Smith DP, Park B, Windham WR (2008) Embedded bone fragment detection in chicken fillets using transmittance image enhancement and hyperspectral reflectance imaging. Sens Instrum Food Qual Saf 2(3):197–207 Google Scholar
- Zhang H, Paliwal J, Jayas DS, White NDG (2007) Classification of fungal infected wheat kernels using near-infrared reflectance hyperspectral imaging and support vector machine. Trans ASABE 50(5):1779–1785 Google Scholar
Author information
Authors and Affiliations
- Department of Biosystems Engineering, University of Manitoba, Winnipeg, MB, R3T 2N2, Canada V. Chelladurai & D. S. Jayas
- V. Chelladurai